Vector Databases: Revolutionizing High-Dimensional Data Management for AI
3 min read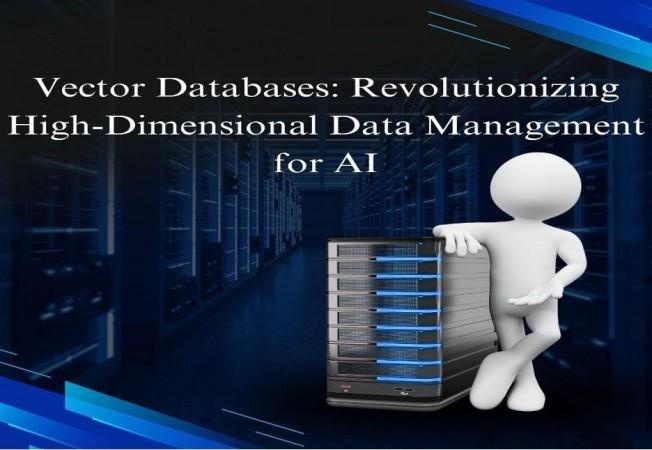
Innovations in database technologies are reshaping the foundations of modern data management. Sandeep Kumar Nangunori, an expert in AI-driven systems, delves into the transformative capabilities of vector databases, highlighting their critical role in managing high-dimensional data for artificial intelligence (AI) applications.
A Paradigm Shift in Data Representation
The growing complexity and diversity of data have led to the transition from traditional relational databases to vector databases. Unlike relational models, vector databases represent data as high-dimensional vectors, effectively capturing semantic relationships and intricate patterns. This makes them particularly well-suited for managing unstructured data such as images, audio, and text embeddings. Vector databases excel in similarity searches, offering enhanced capabilities for recommendation systems, pattern recognition, natural language processing, and other AI-driven applications, driving innovation in data-intensive fields.
Dense, sparse, and hybrid vector representations address diverse data needs effectively. Dense vectors capture detailed features in images or audio, optimizing for rich, high-dimensional data. Sparse vectors, on the other hand, offer memory-efficient solutions for handling extensive datasets. Hybrid models combine these strengths, while advanced indexing techniques combat the “curse of dimensionality,” enabling swift, precise, and scalable data retrieval in complex scenarios.
Scalability and Performance Redefined
Scalability is a hallmark of vector databases. Traditional systems struggle with exponential data growth, particularly with unstructured, high-dimensional formats. Vector databases employ distributed architectures and advanced partitioning strategies to overcome these challenges. Techniques like hierarchical navigable graphs and product quantization enable rapid similarity searches across billions of records.
High-throughput processing harnesses GPU acceleration and parallelization to deliver sub-millisecond query responses, even for massive datasets. Advanced features like intelligent load balancing, caching strategies, and dynamic resource allocation enhance efficiency and scalability, ensuring seamless performance and reliability as data volumes expand exponentially.
Seamless AI Integration
Integration with AI workflows is a major strength of vector databases. They simplify data transformations, feature extraction, and embedding management, enabling seamless updates without requiring full model retraining. Incremental updates to embeddings enhance efficiency, ensuring AI systems remain adaptive and aligned with evolving models and data.
Vector databases also ensure data lineage and reproducibility, crucial for complex AI pipelines. Features like citation models and optimized data loading techniques reduce training times for large-scale models by up to 40%, emphasizing their efficiency.
Transformative Real-Time Applications
Vector databases have revolutionized multiple industries. In e-commerce, they enable real-time recommendation systems by processing user behavior and product embeddings with high accuracy. Visual search capabilities allow customers to find products through image-based queries, enhancing personalization.
In healthcare, these systems facilitate the storage and retrieval of medical imaging data, aiding diagnostics and treatment planning. Personalized medicine benefits from analyzing patient data patterns to recommend tailored treatments.
In finance, vector databases power fraud detection and risk management. Real-time analysis of transactional patterns reduces false positives and enhances anomaly detection. Market analysis tools built on vector databases provide deeper insights into trading patterns and risk profiles, empowering informed decisions.
Challenges and Future Directions
Despite their promise, vector databases face scalability and integration challenges. Distributed systems contend with latency and consistency issues when managing large-scale deployments. Balancing precision and performance is particularly difficult in real-time applications requiring high accuracy.
Future research focuses on self-optimizing systems, advanced indexing techniques, and novel similarity metrics. Quantum computing and adaptive architectures offer solutions for scalability concerns, while standardized interfaces and observability tools aim to simplify AI integration.
As the data landscape evolves, vector databases have become indispensable for managing high-dimensional, unstructured data in AI-driven applications. Sandeep Kumar Nangunori emphasizes their potential to redefine how organizations store, retrieve, and analyze data. With ongoing advancements in scalability, performance, and AI integration, vector databases are poised to play a pivotal role in unlocking the full potential of data-driven innovation.