Ensuring patient safety: The imperative of data quality in healthcare AI – CRN
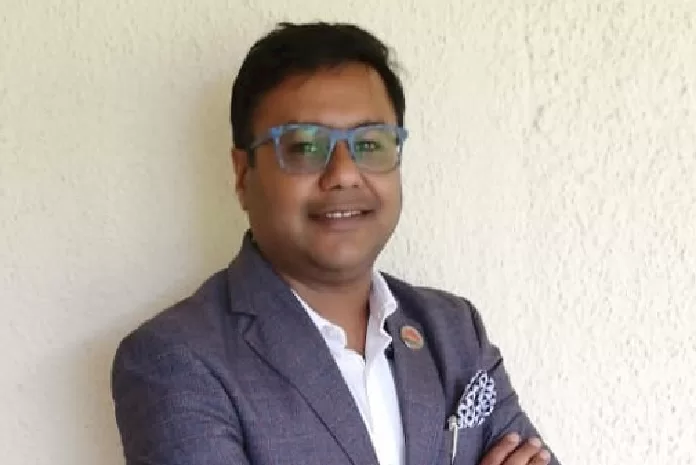

By Mayank Baid, Regional Vice President, India & South Asia, Cloudera
As the use of Gen AI tools in the workplace continues to proliferate and starts becoming a staple much like the document software that we use every day, it follows that many end users trust it to deliver faultless answers. Unfortunately, this assumption does not hold true as recent studies have revealed that Gen AI models often provide answers that are less accurate than its confidence level suggests.
This mismatch between this level of accuracy and the projected confidence of Gen AI tools is especially concerning when we examine the adoption of AI in healthcare, where the stakes are immeasurably higher. Highlighting this concern, an EY report states that 80% of Chief Information Officers (CIOs) in the Indian healthcare industry acknowledge their organisations’ unpreparedness to fully adopt AI technologies.
It behoves healthcare organisations to address inaccuracies in AI models, because what’s at risk is not just efficiency or cost savings to a business, but potentially irreparable harm to human lives, their wellbeing, and their health are on the line.
AI models are as good as the data that is fed to them. This means that AI models used within a healthcare context need to be rigorously tested, provided with reliable data, and supported by robust data management systems to mitigate errors.
Indian enterprises are actively investing in research and development. Projections indicate that AI could contribute USD 25-30 billion to India’s GDP by 2025, significantly enhancing healthcare accessibility, diagnostics, and treatment outcomes. This anticipated growth is bolstered by government initiatives such as the India AI Mission and the Digital Personal Data Protection Act, 2023, which aim to foster a digitally empowered healthcare ecosystem, ensuring responsible AI integration and strengthened data security. Additionally, programs like the Ayushman Bharat Digital Mission (ABDM) are working towards creating an integrated digital health infrastructure, linking practitioners and patients digitally for real-time health records access.
Hallucinations hold immense risk
The challenge with using GenAI built on open-source public LLMs lies in its propensity for hallucinations,errors where the AI generates inaccurate or entirely fabricated information. Despite significant efforts from hyperscalers to address this issue with new products and techniques, none have been able to eliminate hallucinations or guarantee consistent thresholds of accuracy.
While GenAI applications hold great promise, they also carry significant risk. An inaccurate insight derived from GenAI could lead to dire consequences for patient care. GenAI providers shield themselves from liability by including disclaimers in their licensing agreements, cautioning against the use of these tools in high-stakes scenarios. In India, healthcare practitioners are already leveraging GenAI for tasks like interpreting X-rays, MRIs, and CT scans, as well as generating statistical summaries of patient data. This widens the probability of risks wherein erroneous conclusions yielded by GenAI models are mistakenly regarded as accurate. It is thus imperative for healthcare companies to fully recognise these risks and implement rigorous verification processes for any AI-generated diagnosis or recommendation. Responsibility goes beyond ensuring accuracy, it also encompasses the protection of sensitive patient information. Without robust safeguards in place, the use of GenAI may expose data vulnerabilities, including unauthorised access or breaches of confidentiality.
Robust data management mitigates this risk
To address these challenges, developers are exploring ways to improve confidence calibration in GenAI systems. Confidence calibration ensures that the AI system’s reported confidence aligns closely with the actual accuracy of its outputs, reducing the risk of over-reliance on incorrect responses. Achieving this requires three key actions:
- Integrating explicit feedback mechanisms where human users or automated systems provide real-time corrections to the AI.
- Refining training protocols using diverse, representative datasets to minimise biases and improve model robustness.
- Conducting rigorous post-deployment accuracy tests to ensure consistent performance across various conditions.
These efforts depend heavily on access to high quality data, data that is accurate, up-to-date, and diverse. Hybrid data platforms are increasingly becoming the backbone of this process, serving as a single source of truth and enabling organisations to consolidate, validate, and analyse data seamlessly across private data centres, public clouds, and hybrid environments. By providing real-time data access, these platforms serve as foundational enablers for data transformation, implementing feedback mechanisms, refining training, and conducting performance tests.
Features such as robust data cataloguing and lineage tracking enhance transparency by revealing the origin and movement of data, thereby building trust in the insights generated by AI models.
Equally critical is ensuring security and compliance. Hybrid platforms must prioritise robust security practices, including encryption (both at rest and in transit), role-based access controls, and integration with enterprise identity management systems. These measures safeguard sensitive patient information, protect data integrity, and ensure that AI models are trained and operate on secure, trustworthy data. Compliance with regulatory standards further solidifies these safeguards, making hybrid platforms indispensable for mitigating risks in AI-driven healthcare.
The future of healthcare depends on our ability to harness the power of data to improve patient outcomes. By investing in digital healthcare, India can develop a future-ready healthcare system that is resilient, inclusive and capable of adapting to an ever-evolving technological landscape. Embracing digital technologies not only modernises healthcare but positions India as a model for other countries, demonstrating how digital healthcare can improve outcomes, expand access and ultimately create a healthier, more equitable society.