Innovations in Edge AI: Redefining Customer Experience
3 min read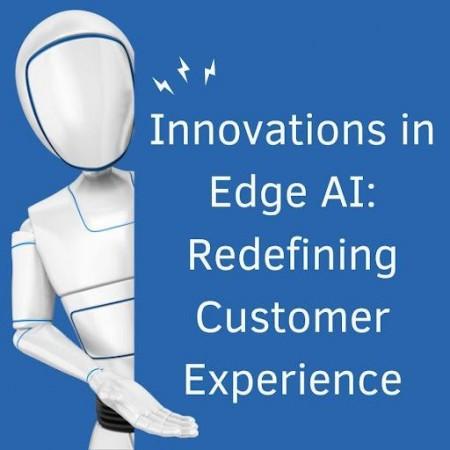
The integration of AI and edge computing is revolutionizing customer interactions across industries. Highlighting this transformative synergy, Mayank Choubey, an accomplished technology innovator, examines how real-time responsiveness, enhanced privacy, and personalized engagements are redefining customer experience (CX). His analysis offers valuable insights into how these advancements are setting new benchmarks for industry excellence and innovation.
The Shift to Localized Data Processing
Edge computing marks a major shift from traditional cloud-based processing by enabling data analysis closer to its source, such as IoT sensors or smart devices. This approach reduces response times and enhances data security, overcoming latency and bandwidth challenges linked to distant data centers. With AI-powered decision-making at the edge, real-time responsiveness is ensured. This localized processing is crucial for industries handling sensitive information, as it bolsters user privacy and meets strict compliance standards.
Optimized AI Models for the Edge
A key challenge in deploying AI at the edge is balancing the need for computational power with the limited resources of many edge devices. Innovations in lightweight AI models have focused on maintaining efficiency without losing accuracy. Techniques like model pruning, quantization, and knowledge distillation help compress AI architectures for smooth execution on low-power devices. Adaptive computation further enables models to adjust complexity based on input and resources, ensuring strong performance across diverse hardware, from powerful edge servers to small IoT devices.
Real-Time Personalization in CX
Edge AI’s main appeal is its potential for real-time personalization. By processing data instantly, businesses can deliver context-aware and tailored experiences aligned with customer behaviors and preferences. In retail, smart mirrors can suggest products based on prior interactions and current choices, while AI-powered kiosks offer quick service and localized recommendations without cloud-related delays. This extends to hospitality and automotive sectors, with in-room voice assistants adapting to guest needs and in-vehicle AI systems providing real-time route updates and personalized entertainment, redefining convenience and user engagement standards.
Enhanced Security and Privacy
Processing data at the edge offers enhanced security by keeping sensitive information local, reducing the risk of breaches and unauthorized access associated with transmitting data to cloud servers. This local approach ensures personal data remains on the device, boosting user trust and compliance with data protection regulations. Additionally, edge devices improve reliability, functioning independently of internet connectivity. This resilience ensures continued operations during network interruptions, making it particularly valuable in environments where uninterrupted service is crucial. The combination of security and reliability enhances overall system performance and trustworthiness.
Challenges and Considerations
Despite its promising potential, implementing edge AI is not without challenges. Hardware limitations are a common hurdle, with edge devices often constrained by limited processing power and memory compared to centralized cloud servers. The deployment of sophisticated AI models on these devices requires careful optimization to strike the right balance between resource efficiency and functional accuracy.
Moreover, integrating edge AI into existing customer experience infrastructures can be complex. Businesses that have invested heavily in cloud solutions must navigate the intricacies of creating a hybrid model that seamlessly connects edge, cloud, and on-premises systems. Effective strategies must ensure consistent data flow and functionality while training staff to manage these new systems.
The Future Landscape
The future of edge AI holds several promising developments. The rise of neuromorphic computing architectures modeled after the human brain could vastly improve the energy efficiency of edge AI systems, enabling more complex algorithms on smaller, more resource-constrained devices.
Additionally, advancements in federated learning will bolster collaborative AI training across distributed edge nodes, ensuring data privacy and improving overall model performance.
Ethical considerations must accompany these technological strides. Ensuring fairness, transparency, and minimizing algorithmic bias are crucial to deploying responsible edge AI solutions. As these systems become integral to daily life, embedding strong ethical practices into their design and implementation will be vital.
In conclusion, exploring edge AI underscores a transformative phase in customer experience. Mayank Choubey illustrates how real-time responsiveness, security, and personalization blend to create seamless, intuitive, and private interactions. As industries adopt these advancements, customer engagement standards will be redefined, offering tailored, context-aware experiences that align with modern user expectations. This evolution marks a new era where businesses can effectively meet customer needs.