Latest Aerospike Vector Search keeps data fresh for accurate GenAI and ML decisions regardless of scale – CRN
3 min read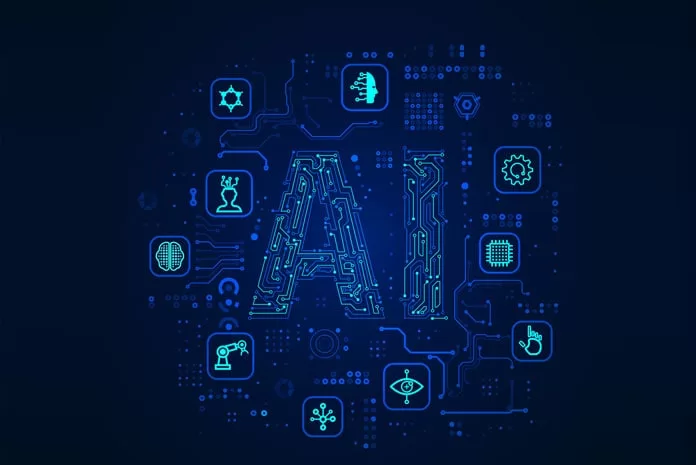
Aerospike Inc. unveiled the latest version of Aerospike Vector Search featuring powerful new indexing and storage innovations that deliver real-time accuracy, scalability, and ease-of-use for developers. These advancements simplify deployment, reduce operational overhead, and enable enterprise-ready solutions for just-in-time generative AI (GenAI) and ML decisions.
One of the three most popular vector database management systems on DBEngines, Aerospike unlocks real-time semantic search across data, delivering consistent accuracy no matter the scale. It lets enterprises easily ingest vast amounts of real-time data and search billions of vectors within milliseconds—all at a fraction of the infrastructure costs of other databases.
Durable self-healing indexing
The latest release of Aerospike Vector Search adds a unique self-healing hierarchical navigable small world (HNSW) index. This innovative approach allows data to be ingested immediately while asynchronously building the index for search across devices, enabling horizontal, scale-out ingestion. By scaling ingestion and index growth independently from query processing, the system ensures uninterrupted performance, fresh, accurate results, and optimal query speed for real-time decision-making.
Flexible storage
Aerospike’s underlying storage system also provides a range of configurations to meet customers’ needs in real time, including in-memory for small indexes or hybrid memory for vast indexes that reduces costs significantly. This unique storage flexibility eliminates data duplication across systems, management, compliance and other complexities.
“Companies want to use all their data to fuel real-time AI decisions, but traditional data infrastructure chokes quickly, and as the data grows, costs soar,” said Subbu Iyer, CEO, Aerospike. “Aerospike is built on a foundation proven at many of the largest AI/ML applications at global enterprises. Our Vector Search provides a simple data model for extending existing data to take advantage of vector embeddings. The result is a single, developer-friendly database platform that puts all your data to work—with accuracy—while removing the cost, speed, scale and management struggles that slow AI adoption.”
Easily start, swap, and scale AI applications
Application developers can easily start or swap their AI stack to Aerospike Vector Search for better outcomes at a lower cost. A new simple Python client and sample apps for common vector use cases speed deployment. Developers can also add as many vectors as they want to existing records and AI applications with the Aerospike data model.
Aerospike Vector Search makes it easy to integrate semantic search into existing AI applications through integrations with popular frameworks and provider cloud partners. A Langchain extension speeds the build of RAG applications, and an AWS Bedrock sample embedding example speeds the build-out of your enterprise-ready data pipeline.
Multi-model, multi-cloud database platform
Aerospike’s multi-model database engine includes document, key-value, graph, and vector search all within one system. This significantly reduces operational complexity and cost and lets developers choose the best data model for each specific application use case. Aerospike’s graph and vector databases work independently and jointly to support AI use cases, such as retrieval augmented generation (RAG), semantic search, recommendations, fraud prevention, and ad targeting. The Aerospike multi-model database is also available on all major public clouds, giving developers the flexibility to deploy real-time applications wherever and however they like, including in hybrid environments.