Risk Management Expert Nitesh Khullar Drives ML/Al Adoption in Credit Modeling
4 min read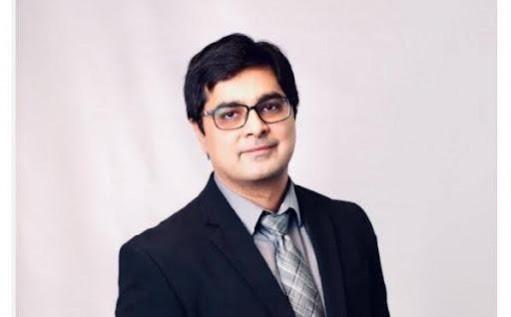
“The future of credit risk modeling is, undeniably, in the hands of artificial intelligence,” declares Nitesh Khullar, senior vice president of scoring, modeling & strategic analytics at an international banking firm. “Financial risk assessment doesn’t need to be subject to human error or bias any longer. Now, complex neural networks can offer precise, rapid logic at the push of a button.”
Nitesh Khullar is a seasoned risk management leader with over 15 years of leadership and coaching experience within banking and financial services. He specializes in end to end risk management, fraud risk management and strategic analytics across lending products, with expertise in data analytics and risk modeling.
Khullar’s bold statement comes at a rather exciting moment in the banking industry. As financial institutions ready themselves for economic uncertainties and a new wave of technologies, integrating ML/AI into credit risk management appears to be a universal concept. With over 15 years of experience in the field, Khullar has been tasked with steering one of the world’s largest banks through the fog of ML/AI adoption.
The Promise of AI in Credit Modeling
Khullar’s team at Citibank is among the first in this regard, developing sophisticated ML/AI-driven credit scoring models that promise to alter how banks assess creditworthiness entirely. Khullar’s recent ML risk scoring model has proven to be very effective in managing the risk exposure of business while focusing on the growth. This model was greatly appreciated within his financial institution and has set standards for the business.
“We’re moving beyond traditional credit scores,” Khullar explains. “Our ML/AI models can analyze vast amounts of alternative data, from social media activity to transaction histories, providing a more complete view of an applicant’s financial health.”
This movement toward ML/AI-powered credit assessment is not without its challenges, however. Critics argue that such models risk perpetuating biases and lack the transparency required by regulatory bodies.
Khullar himself acknowledges these concerns but maintains his stance on its use. “Explainability is a top priority for us,” he asserts. “We’re investing heavily in techniques like SHAP (SHapley Additive exPlanations) to make our ML/AI models more interpretable. That way, we can provide clear rationales for credit decisions and make sure that no biases are present.” Khullar’s expertise in data science and machine learning data modeling led to development of SHAP based reject reasons algorithm and is being currently adopted across business within his financial institution.
The numbers themselves tell the story of an industry on the brink of upheaval. According to a recent report by Grand View Research, the global market for generative AI in financial services is projected to grow at a staggering compound annual growth rate of 39.1% from 2024 to 2030, reaching an estimated $16.02 billion by the end of the decade. A conservative one, by the estimates of some.
The Road Ahead: Balancing Development and Regulation
As banks like Khullar’s forge ahead with AI adoption, regulators are scrambling to keep pace. In 2023, the Federal Reserve, along with other financial regulators, issued joint guidance on ML/AI use in banking, proclaiming the need for extensive risk management frameworks and ongoing monitoring of AI systems.
Khullar sees this regulatory scrutiny as an opportunity rather than a hindrance. “Clear guidelines will actually help speed up ML/AI adoption by giving us a framework for responsible development. It’s about finding the right balance between pushing the boundaries and keeping the ethics fundamental to our industry,” he argues.
Khullar sees a future where ML/AI enhances credit decisions and greatly aids in detecting fraud and managing systemic risks. A report by McKinsey & Company supports this idea, estimating that ML/AI could potentially add between $200 billion and $340 billion in annual value to the banking sector if fully implemented across various use cases. Khullar’s team developed ML based fraud tools which is helping to identify and evaluate the fraud behavior of applicants and significantly reduced fraud exposure of business.
The Human Element in an ML/AI-Driven World
Despite the speed of development seen with ML/AI technologies, Khullar explains that human skill remains irreplaceable. “ML/AI is a powerful tool, but it’s not a magic wand. The real value comes from combining AI-specific tasks with human judgment.”
Such a perspective is echoed in the broader machine learning/artificial intelligence sphere. While ML/AI is expected to automate many aspects of financial services, a survey by the World Economic Forum predicts that 133 million new roles may emerge by 2025 as a result of the division of labor between humans, machines, and algorithms.
With change being the only constant in modern finance, Nitesh Khullar and his team are betting big on ML/AI–expecting it to be the next development in credit risk technology. However, the true test will lie in using its power responsibly, ascertaining that the technology serves not just the bottom line but the very human lives relying on it.