Advancing Cybersecurity: How AI is Revolutionizing Threat Modeling
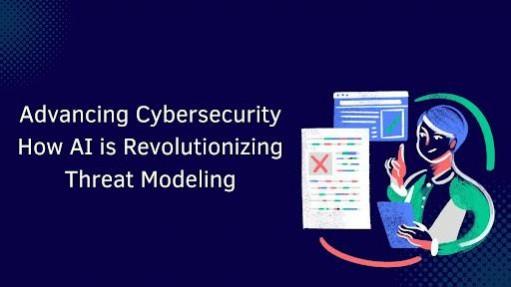
Artificial intelligence (AI) is rapidly reshaping the cybersecurity landscape, bringing unprecedented efficiency and accuracy to threat modeling. Bhooshan Ravikumar Gadkari, an expert in the field, explores how AI-driven techniques are enhancing security strategies by identifying risks faster and more effectively than traditional methods. His insights provide a deep dive into AI’s role in improving cybersecurity preparedness.
The Evolution of Threat Modeling
Threat modeling has been fundamental to proactive cybersecurity, enabling organizations to identify and mitigate risks before breaches occur. Traditional methods depend on human expertise to assess systems and define vulnerabilities, but growing digital complexity makes manual approaches insufficient. AI-driven threat modeling revolutionizes this field by automating risk detection, analyzing vast data sets, identifying anomalies, and predicting attack vectors with superior speed and accuracy, enhancing security teams’ effectiveness.
AI-Powered Tools in Cybersecurity
The integration of AI into threat modeling has led to the development of advanced tools designed to enhance security protocols. One such innovation is the application of large language models to identify and categorize potential threats efficiently. AI-driven frameworks leverage historical threat data, security logs, and vulnerability databases to continuously refine risk assessments.
Additionally, AI-powered tools enable the mapping of emerging threats to global cybersecurity frameworks, allowing security teams to preemptively respond to new attack methods. By utilizing machine learning, these systems can provide organizations with real-time updates on evolving threats, improving their ability to prevent cyberattacks.
Benefits of AI in Threat Modeling
AI enhances cybersecurity in several ways, primarily by improving efficiency, accuracy, and scalability.
- Speed and Automation: Traditional threat modeling requires extensive human effort to analyze risks, but AI systems can process large-scale data in real time, accelerating security assessments.
- Enhanced Accuracy: AI algorithms identify patterns that human analysts might overlook, reducing errors and improving the precision of risk assessments.
- Scalability: AI-powered tools can be deployed across vast digital ecosystems, ensuring that security measures remain consistent even in complex networks.
- Up-to-Date Threat Intelligence: AI continuously learns from new cyber threats, providing organizations with updated insights to mitigate risks more effectively.
These benefits make AI an indispensable tool in modern cybersecurity frameworks, equipping organizations with a proactive defense mechanism against sophisticated cyber threats.
Challenges of AI-Driven Security
While AI-driven threat modeling offers significant benefits, it also presents challenges that organizations must address. Data quality is a major concern, as AI models depend on large datasets, and any bias or inaccuracies can lead to flawed security predictions. Additionally, AI-generated risk assessments often function as “black boxes,” making interpretation difficult and affecting trust. Over-reliance on AI may also diminish the value of human expertise. Balancing AI automation with human oversight is essential for a robust cybersecurity strategy.
The Future of AI in Cybersecurity
The future of AI-driven threat modeling is set for major advancements, with technologies like deep learning and reinforcement learning enhancing predictive capabilities, allowing organizations to anticipate cyber threats before they occur. A key development is AI’s integration with anomaly detection systems, enabling real-time identification of deviations from normal network behavior and flagging potential security breaches. These AI models will continuously learn from new attack patterns, improving their ability to detect and prevent threats. Additionally, AI-human collaboration will strengthen, with enhanced explainability features allowing security professionals to interpret AI-generated risk assessments more clearly. This fusion of AI automation and human expertise will create more resilient and effective cybersecurity defenses against evolving digital threats.
In conclusion, Bhooshan Ravikumar Gadkari emphasizes that AI-driven threat modeling significantly enhances risk identification and mitigation. However, addressing challenges like data quality, interpretability, and automation reliance is crucial. By integrating AI with human expertise, organizations can build resilient security frameworks, ensuring proactive defenses as AI continues to shape the future of cybersecurity strategies worldwide.