Balancing AI Costs and Performance with Hybrid Infrastructure
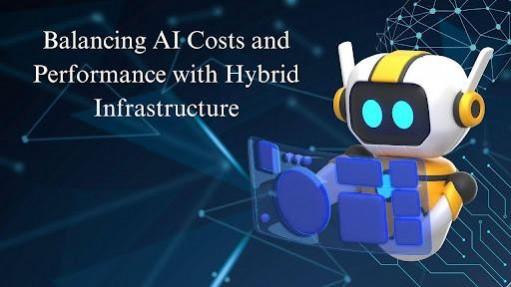
Artificial Intelligence (AI) has become a cornerstone of modern business, yet managing its escalating costs remains a persistent challenge. Arthi Rengasamy, an independent researcher, presents an in-depth analysis of how hybrid infrastructure optimizes AI’s Total Cost of Ownership (TCO). Her study highlights the innovations that enable organizations to achieve cost efficiency without compromising performance.
The Growing Burden of AI Infrastructure Costs
The rapid adoption of AI technologies has significantly increased computational demands, with AI model training requirements growing by 312% from 2018 to 2024. This surge has stressed traditional infrastructure models, as organizations relying exclusively on cloud resources often face unpredictable costs. On the other hand, those using on-premises solutions experience scalability challenges. Hybrid infrastructure, which combines both cloud and on-premises environments, provides a cost-effective solution. It allows organizations to optimize resources, balance performance and cost, and scale more efficiently, offering the flexibility to meet growing AI demands without overburdening any single infrastructure model.
Strategic Workload Distribution for Cost Optimization
A key benefit of hybrid infrastructure is its ability to strategically allocate workloads based on their requirements. For AI training, which demands substantial computational power, deploying on-premises can reduce cloud costs by up to 80%. This helps manage expenses while meeting the heavy processing needs. On the other hand, experimental workloads, which require flexibility and scalability, can be managed using cloud resources, cutting development cycle times by 45%. By combining on-premises and cloud solutions, organizations can optimize resource use, ensuring both cost-efficiency and high performance while maintaining peak operational efficiency
Enhancing AI Model Inference with Hybrid Strategies
AI inference workloads, where trained models generate real-time predictions, greatly benefit from hybrid deployment. By processing time-sensitive data on-premises, organizations can ensure quicker decision-making and minimize latency. Less critical tasks can be offloaded to the cloud, lowering operational costs by up to 40%. This strategic division of labor not only reduces costs but also improves latency by 45%, allowing AI-driven applications to remain highly responsive and efficient, ensuring optimal performance while managing resource utilization effectively.
Optimizing Data Storage for Cost and Compliance
Data management remains a crucial component of AI infrastructure. Organizations using hybrid storage strategies achieve 47% better cost efficiency while maintaining compliance with data sovereignty regulations. Sensitive data can be stored on-premises, while non-sensitive datasets leverage cost-effective cloud storage, reducing overall storage expenses by 25-35%.
Hybrid Deployment for High-Risk AI Applications
Industries handling high-risk workloads, such as finance and healthcare, require stringent security and compliance measures. Hybrid infrastructure provides a tailored solution by keeping mission-critical workloads on-premises while using the cloud for secondary tasks. This approach improves security compliance scores by 51% and reduces regulatory costs by 43%.
Business Continuity and Disaster Recovery in AI Operations
AI-driven organizations must ensure uninterrupted service availability. Hybrid infrastructure enhances disaster recovery by offering a balance between cloud-based failover systems and on-premises redundancy. Companies adopting this model report a 45% improvement in recovery time objectives (RTO) and achieve cost savings of up to 42% in disaster recovery expenses.
Technology Management for Smarter Cost Control
Sophisticated cost management frameworks are essential for sustainable AI adoption. Organizations implementing real-time monitoring and automated scaling solutions achieve a 50% reduction in unnecessary infrastructure expenses. AI-powered optimization tools further improve resource utilization, leading to an overall infrastructure cost reduction of 35%.
In conclusion, hybrid infrastructure has emerged as a transformative solution for optimizing AI’s TCO while maintaining operational efficiency. By strategically distributing workloads, optimizing storage, and ensuring business continuity, organizations can navigate AI’s cost challenges without sacrificing performance. Arthi Rengasamy‘s insights reinforce that as AI adoption accelerates, a hybrid model will be essential for sustaining innovation and financial efficiency. The flexibility of this approach ensures that organizations can meet the growing demands of AI technologies while managing costs effectively, driving both performance and financial sustainability.