Revolutionizing Healthcare with Edge-Enhanced Federated Architecture
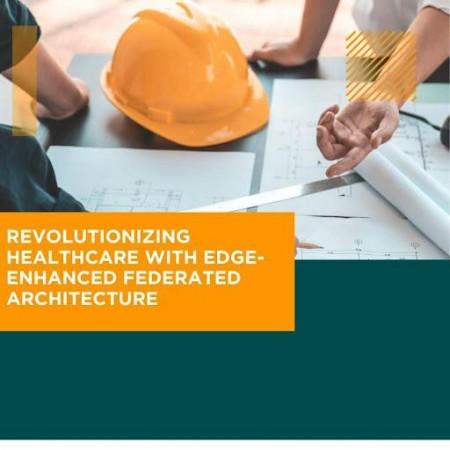
In an era of digital transformation, healthcare is leveraging technological innovations to enhance patient monitoring while ensuring data privacy. Bharath Kumar Reddy Janumpally presents a pioneering approach integrating edge computing with federated learning, reinforced by a zero-trust security framework. This novel architecture revolutionizes real-time patient monitoring by optimizing efficiency, strengthening security, and ensuring regulatory compliance, setting a new benchmark for modern healthcare solutions.
The Need for Smarter Healthcare Solutions
The rise of connected medical devices has enabled continuous health monitoring, yet existing cloud-based architectures struggle with latency and data security challenges. Traditional systems centralize data processing, making them vulnerable to privacy breaches and operational inefficiencies. The proposed Edge-Enhanced Federated Cloud Architecture (EEFCA) addresses these issues by shifting computation closer to the data source while ensuring patient information remains secure.
Edge Computing: Bringing Processing Closer to the Patient
Edge computing is at the heart of this innovation, enabling real-time data processing near the point of collection. Medical IoT devices, such as wearable monitors, transmit data to local edge nodes where initial processing occurs. This reduces latency and enhances responsiveness, crucial for critical care scenarios. By analyzing data locally, unnecessary transmissions to central servers are minimized, improving efficiency and security.
Federated Learning: Advancing AI Without Compromising Privacy
The federated learning framework revolutionizes healthcare AI by allowing collaborative model training across multiple facilities without sharing raw patient data. Each healthcare node trains AI models locally, contributing anonymized updates to a global model. This distributed learning approach ensures improved diagnostic accuracy while maintaining patient confidentiality. The use of differential privacy techniques further strengthens data protection, making it resilient against unauthorized access.
Zero-Trust Security: A New Standard for Healthcare Data Protection
With cyber threats on the rise, conventional perimeter-based security models are insufficient for safeguarding sensitive health information. The zero-trust framework incorporated into this architecture mandates continuous verification for all access requests. Multi-factor authentication, role-based controls, and behavioral analytics collectively reinforce security, ensuring that only authorized personnel can access critical data. Additionally, automated threat detection mechanisms proactively mitigate risks in real time.
Real-World Application: Remote Patient Monitoring
A key application of this architecture is in remote patient monitoring systems. By leveraging IoT-enabled medical devices, real-time health assessments become feasible, even for patients in remote locations. Secure data transmission, edge-based analytics, and federated learning combine to deliver insights without violating privacy regulations. This approach not only enhances patient care but also ensures compliance with stringent data protection laws.
Performance and Privacy: Striking the Right Balance
Experimental evaluations demonstrate a 65% latency reduction over traditional cloud-based solutions. Federated learning and differential privacy measures protect patient data during AI training, ensuring no individual data exposure. Zero-trust security implementation effectively blocks unauthorized access attempts while maintaining seamless usability for healthcare professionals. This approach enhances security and privacy while delivering efficient real-time monitoring solutions, setting a new benchmark for the future of healthcare technology.
Overcoming Challenges and Future Prospects
Despite its advantages, the architecture faces challenges related to edge device processing limitations and integration with legacy healthcare systems. Future improvements could include optimizing federated learning algorithms for resource-constrained devices and enhancing interoperability between modern and existing medical infrastructures. Additionally, emerging technologies such as quantum-resistant cryptography and explainable AI could further refine security and transparency.
In conclusion, Bharath Kumar Reddy Janumpally‘s work establishes a foundation for scalable, privacy-centric healthcare solutions, setting a new standard in patient data security and real-time monitoring. This innovative framework presents a promising path for the future of healthcare monitoring, ensuring real-time responsiveness, privacy preservation, and robust security. As digital health solutions continue to evolve, integrating advanced technologies like edge computing, federated learning, and zero-trust security will be pivotal in shaping a more secure and efficient healthcare ecosystem.